Finding the Horn in the Haystack
Machine learning and tech tools help officials narrow efforts to catch wildlife traffickers
ADF STAFF
Twelve years ago, Dr. Andrew Rhyne, a marine biologist and expert on wildlife trafficking, was trying to figure out a better way to track the aquarium fish being imported into the United States.
The system could not keep up with the trade volume. About 130 Fish and Wildlife Service officers had to inspect hundreds of thousands of shipments of all types of animals. Much of it required manually cross-referencing information on a shipping document with people or businesses on blacklists for previous offenses. There also was the matter of making sure that the fish in the shipment matched what was declared. With about 40,000 marine species to keep track of, this was no easy task.
“There are so many things coming across borders, and inspectors don’t have time,” Rhyne told ADF. “We’re asking them to know everything about everything and try to find the illegal stuff that’s in there.”
Rhyne and his co-creators developed the Nature Intelligence System (NIS), which digitizes documents and uses algorithms to examine buyer and seller histories. It cross-references them against people with criminal backgrounds and examines numerous other pieces of data to make sure shipments are likely to be the correct species, quantity and actually are coming from the country they claim to be from.
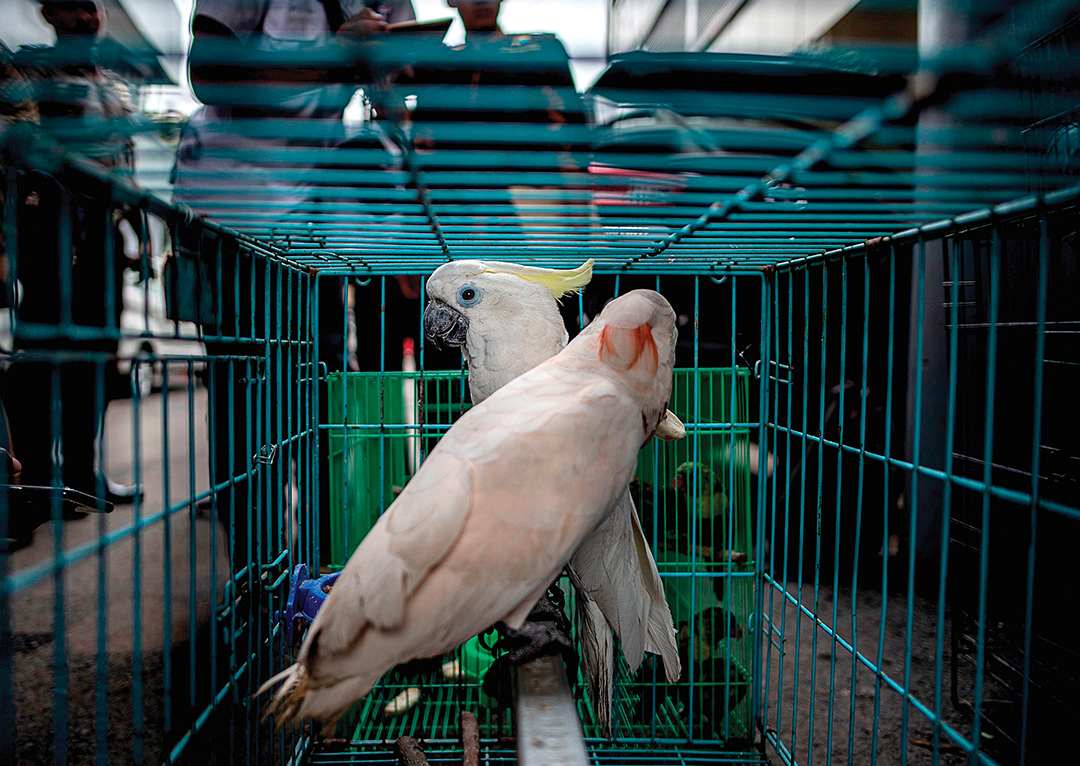
“If you have this massive volume of information, you’re basically trying to find the needle in the haystack,” Rhyne said. “So our system essentially was built to alleviate the bulk of the paperwork that agencies are doing right now.”
The system helps agencies target their limited resources by inspecting only the shipments most likely to be illegal. This is important since, on average, inspectors have time to check only about 10% of all shipments that pass through customs. London Heathrow Airport alone processes 28 million live animals each year.
Traffickers take advantage of this lack of capacity to move live animals or animal parts without making much effort to hide them. They believe the odds are on their side.
“The agents get so many things in, and we’re asking them to identify 20,000 species of things and derivatives,” Rhyne said. “It’s so unfair to ask a human to know all that stuff, so the computer just kind of does that for them.”
The system won the Wildlife Crime Tech Challenge in 2016 given by the U.S. Agency for International Development. Rhyne hopes it can be adopted widely. NIS is just one way law enforcement agencies are gaining the upper hand on criminals through the use of machine learning and other technology.
Scanning Social Media
Illegal wildlife trafficking occurs openly on social media. Although most prominent social media sites now outlaw animal sales, deals continue to be arranged there and traffickers quite brazenly display their activity.
A 2020 study by the Alliance to Counter Crime Online found that, despite restrictions, traffickers continue to sell hundreds of millions of dollars’ worth of live animals or parts on Facebook. The alliance said 58% of Facebook pages engaged in the illegal wildlife trade clearly used the words “buy,” “sale” or “sell.”
Machine learning can help identify these perpetrators.
A study by Finnish researchers Enrico Di Minin and Christoph Fink showed that algorithms can search for key terms, people or even recognize specific species in photos and videos posted on social media. Programs can be made to recognize animals by the sounds they make on videos.
“The whole system can be automated so that data are mined directly from social media platforms,” the authors wrote. “The content is filtered and only relevant content is kept for further investigations by a computer or a person.”
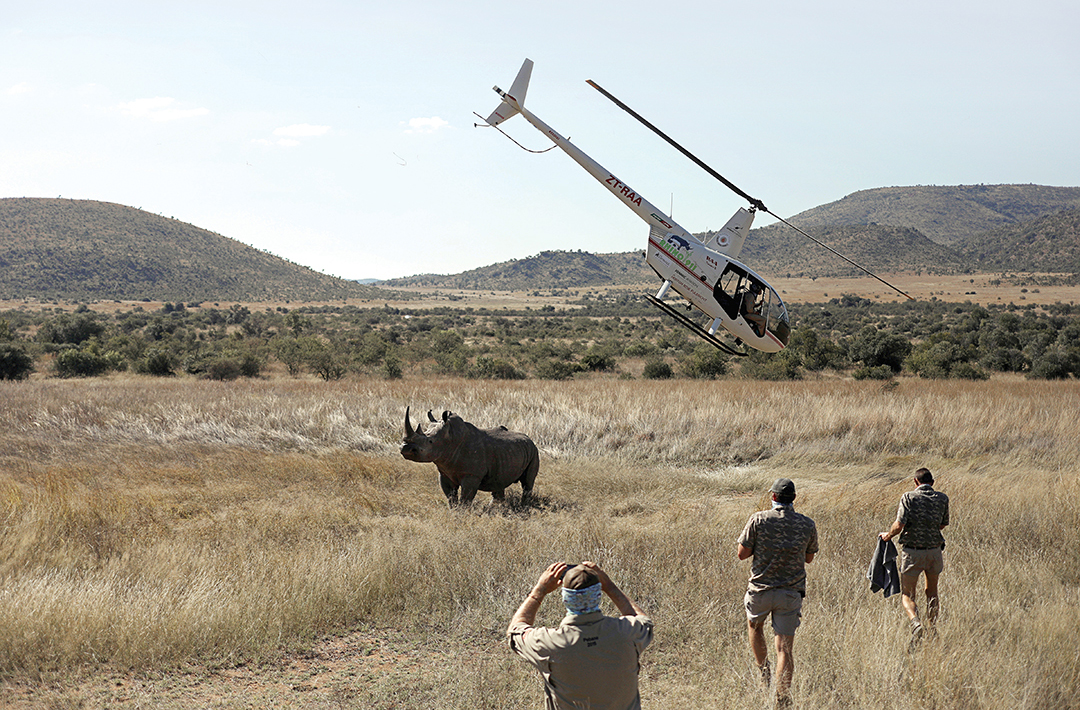
Machine learning systems also can be programmed to identify traffickers’ code words and suspicious travel patterns. For instance, if a person is found on social media to be moving back and forth between trafficking hot spots, that can be flagged for further investigation.
Since traffickers tend to associate with one another, developers can create an algorithm to analyze every photo of a suspected trafficker to gather contact information. Social media profiles offer a treasure trove of information, including locations, methods and phone numbers.
“People involved in the wildlife trade very often have their Facebook profiles open and openly show off their wealth,” Stephen Carmody, chief investigator of Wildlife Justice Commission, told The Independent, a newspaper in the United Kingdom. “They are operationally very poor. They don’t change their phone numbers regularly, they meet customers at the same bars or restaurants and they don’t practice surveillance. Rather than organised crime, I would call [wildlife criminals] disorganised crime.”
Better Optics
Advances in camera technology mean law enforcement agencies have the ability to surveil borders, roads and nature reserves. But the question remains: How can investigators sort through and categorize this imagery to find valuable data to stop criminals?
One answer comes in the form of a camera the size of a pencil backed by machine learning. The nonprofit RESOLVE developed TrailGuard AI in partnership with the tech company Intel. The camera feeds video to a system that learns to identify animals, flags unknown people and captures other poaching signs.
During a field test at the Grumeti Reserve in Tanzania, TrailGuard helped authorities arrest 30 poachers and seize more than 589 kilograms of bushmeat. The team hopes to expand it to be used in 100 game reserves across the continent.
“Large park boundaries and rough terrain mean that rangers often only find out about poaching when it’s too late,” Eric Dinerstein, RESOLVE’s director of wildlife solutions, told Al-Jazeera. “TrailGuard acts as an early warning system, transitioning ranger teams into fully mobile, rapid-response units so that they can respond to would-be poachers and stop them in their tracks.”
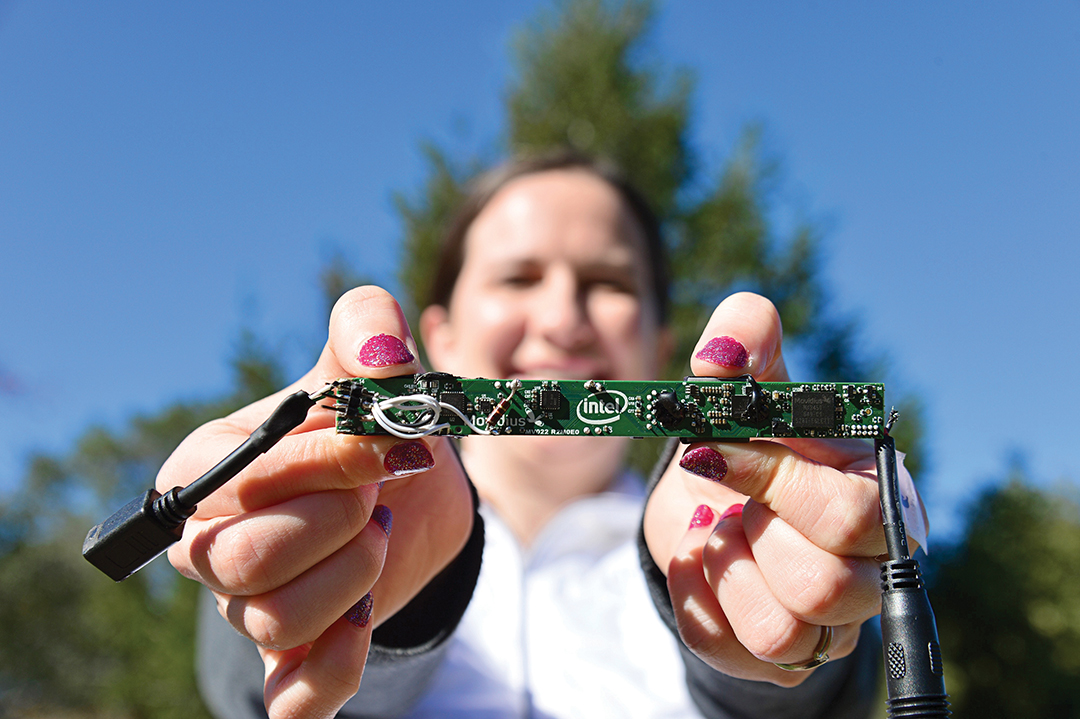
As programs advance they can be programmed to recognize things such as animal carcasses or poachers’ snares. Some systems are developing “predictive” technology that incorporates reams of data to predict where a poacher might strike next.
“Image recognition algorithms can quickly analyse the vast amount of optical data coming in from surveillance streams and give teams an early warning of any threats so they can respond,” the Royal Society wrote in the report “Science: tackling the illegal wildlife trade.” “The algorithms can differentiate between wildlife and human presence. This helps small teams understand where the wildlife is positioned and potential intrusions so they can target resources effectively.”
Machine learning also has enormous possibilities at airports and border crossings, making it possible to sort through X-ray images already captured to look for wildlife parts such as bones.
A project led by researchers at the University of New South Wales in Australia was able to use X-ray technology to differentiate between quills on a domestically bred and wild animal known as an echidna. Other technology is being developed to identify items such as rhino horn or ivory when it appears on an X-ray.
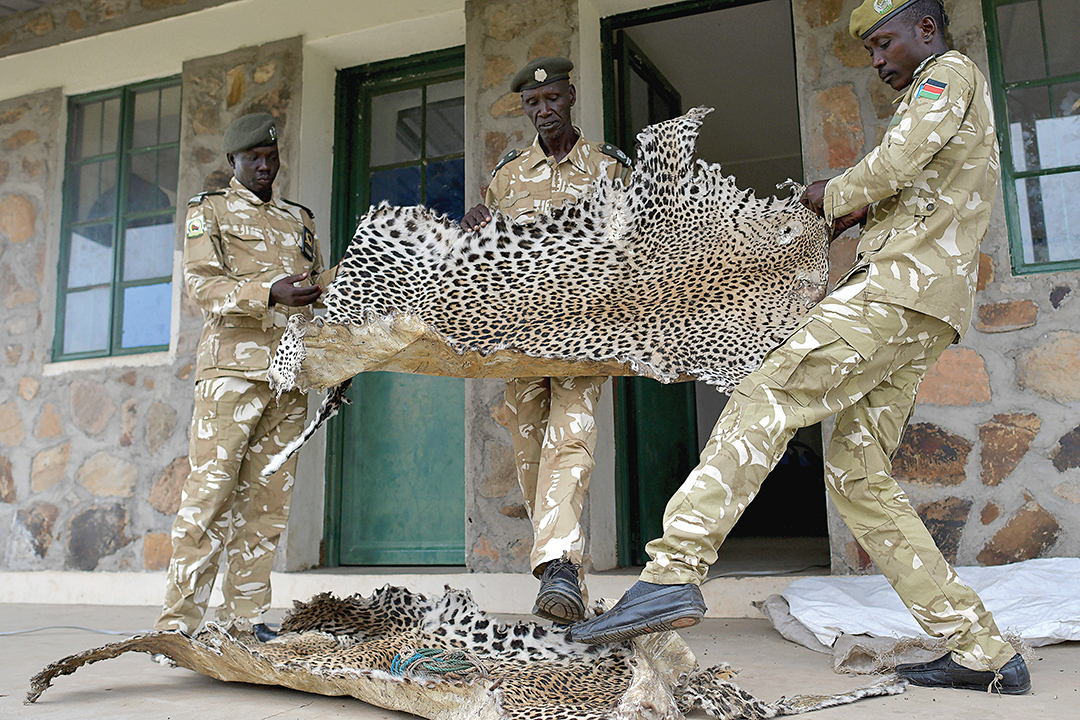
Rhyne said there is now a global recognition that border agents and park rangers are overwhelmed by the scope of the trafficking problem. “We put an undue burden on wildlife inspectors and regulatory agencies,” he said. “They’re not equipped to handle what is coming across borders.”
He said it is time to use machine learning as a way to fight back. He encouraged all countries, no matter their financial constraints or technological limitations, to seek out partnerships that will let them use technology to combat wildlife trafficking. There are companies and groups eager to join forces to address this global problem. “There is enough will in the world to fund it and to do it,” Rhyne said.
How Machine Learning Works
Programmers create an algorithm, or a set of rules, that teaches a machine to recognize an image, word, sound or other important detail. As the machine receives more information and a human expert manually identifies which pieces of data, like a photo, are significant and which are not, the machine can improve its ability to identify important things. It can sort through video or webpages faster than a human, saving time. It also can be programmed to catch details imperceptible to the human eye.
Uses to Stop Wildlife Crime
Social Media: Algorithms can search social media platforms for key words or images related to trafficking, poaching and the sale of wildlife online.
Surveillance: Cameras paired with machine learning software can spot poachers, vehicles and animals in wildlife reserves, allowing officials to respond faster. They also can detect gunshots and other sounds.
Enforcement: Border control officials can upload customs documents to programs, and algorithms will cross-check to make sure there are no “red flags.” This means checking to see whether sellers are blacklisted, ensuring that the country of origin is correct, and confirming details to help officers determine that the animals being shipped match the animals on the paperwork.
Comments are closed.